Imagine stepping into a building where the temperature feels like it was custom-made for you—not based on a generic standard, but perfectly aligned with your preferences, activity level, and even how you’re feeling that day. This isn’t science fiction; it’s the emerging reality powered by the intersection of machine learning and building automation.
The Nuance of Human Comfort
To grasp why this technological leap is so impactful, it’s important to first understand the complexity of human thermal comfort. Temperature is deeply personal. A programmer seated quietly at their desk might crave a cooler breeze, while a maintenance worker navigating a mechanical room might appreciate a warmer climate. Traditional HVAC systems, built around broad averages, have always wrestled with this inherent diversity.
The Shortcomings of One-Size-Fits-All HVAC
Conventional climate control systems operate like a sledgehammer in a world that needs a scalpel. One thermostat often governs vast spaces, resulting in a climate that’s acceptable for some but perfect for no one. It’s an everyday struggle: someone’s too cold, someone else is too hot, and everyone’s productivity takes a hit. Personal heaters, desk fans, and constant manual adjustments become the norm, driving up energy waste and frustration.
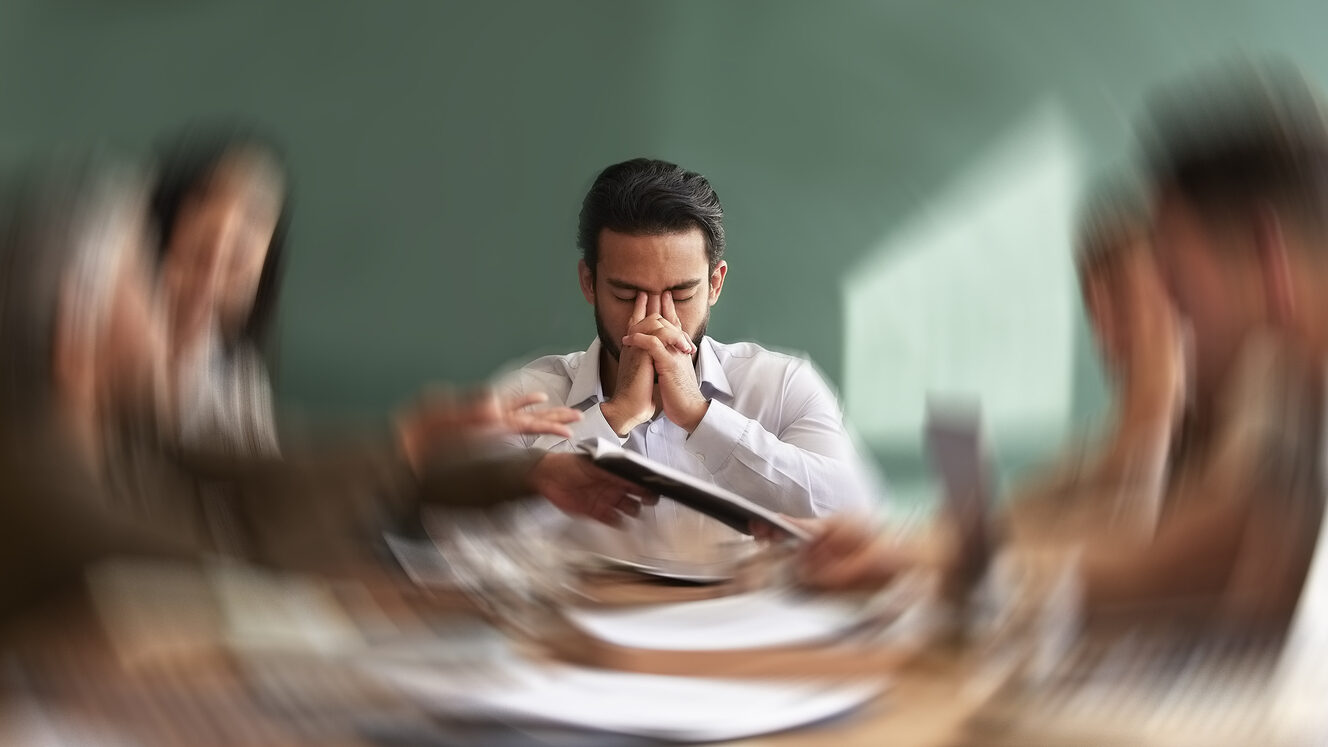
Enter Machine Learning: A Smarter Way to Stay Comfortable
Machine learning flips the script by transforming temperature control into a precise, adaptive art. These advanced algorithms don’t just manage HVAC systems—they learn. By analyzing a myriad of data points, they continuously refine their approach to deliver an environment that works for everyone.
How Does Machine Learning “Learn” Comfort?
Think of machine learning algorithms as hyper-observant assistants. Like an intuitive host at a dinner party, they pick up on cues—subtle or explicit—over time. Here’s how they do it:
1. Physical Environment Data:
- Room temperature
- Humidity
- Airflow
- Light levels (natural and artificial)
- Outdoor weather
2. Individual Preferences:
- Clothing (heavier jackets vs. light blouses)
- Activity level (sitting at a desk or actively moving around)
- Historical comfort preferences
- Physiological factors like age or metabolism
3. Contextual Insights:
- Time of day
- Occupancy levels
- Room usage patterns
- Event schedules (e.g., a meeting room filled with people generates more heat)
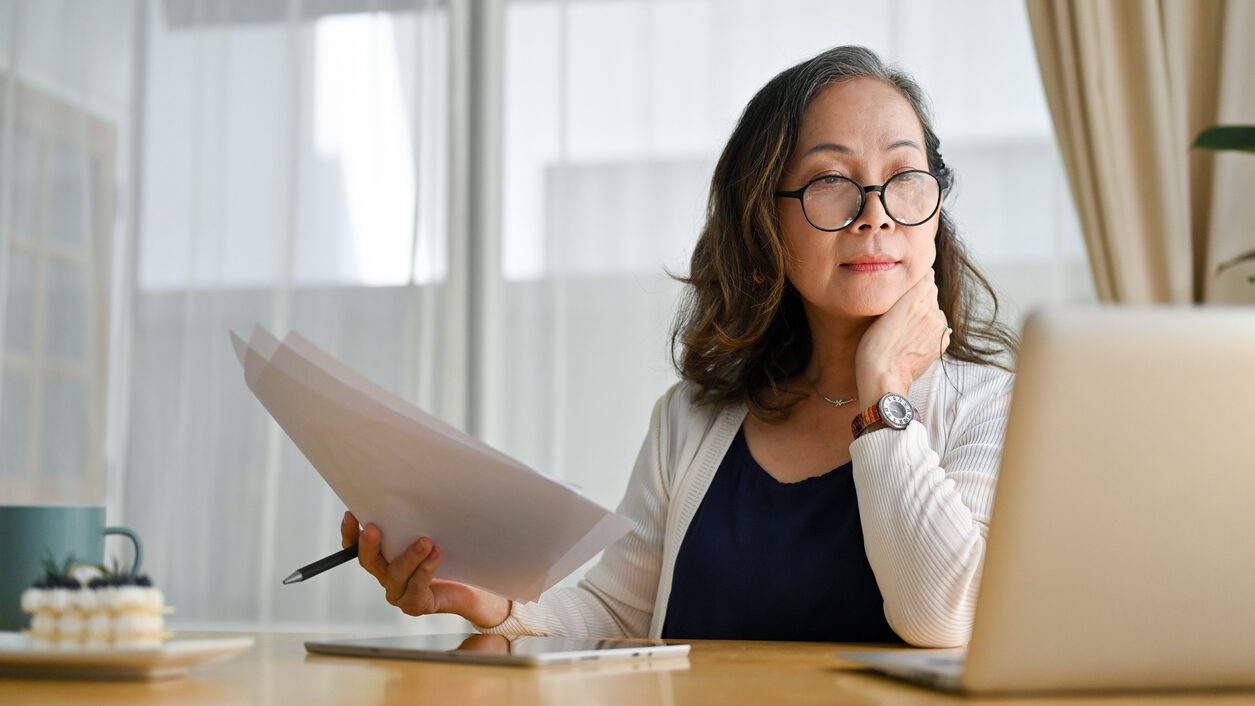
Refining Comfort Over Time
In practice, machine learning begins with educated guesses. It adjusts temperatures, collects feedback—whether explicit, like manual thermostat changes, or subtle, like increased movement in discomfort—and iterates. Over time, the system becomes astonishingly accurate, proactively managing comfort with minimal input.
This isn’t just about keeping everyone cozy. The ripple effects of machine learning in building environments are profound:
1. Energy Efficiency:
Tailored climate control eliminates the overcooling or overheating of unoccupied spaces. Studies show energy savings of up to 30%, directly reducing operational costs and environmental impact.
2. Enhanced Productivity and Well-being:
Comfortable employees are happier and more productive. Research consistently links optimized thermal conditions to better focus, reduced stress, and improved overall satisfaction.
3. Sustainability at Scale:
Energy efficiency translates to a smaller carbon footprint. Machine learning doesn’t just optimize buildings; it helps meet critical ESG goals by lowering emissions without compromising occupant experience.
Challenges and Ethical Dilemmas
As promising as it sounds, this approach comes with strings attached. Data privacy is a top concern. Gathering personal comfort metrics—whether through sensors or wearables—requires robust, transparent policies. Opt-in systems, anonymization, and clear communication are essential to build trust and maintain compliance with privacy regulations.
The Future of Machine-Learning-Driven Comfort
The next generation of these systems will push boundaries even further. Picture a building that:
- Syncs with wearables to track health metrics like heart rate and skin temperature.
- Predicts comfort needs based on individual health and environmental changes.
- Adapts instantly to shifts in weather or occupancy.
This evolution promises a seamless, personalized experience where buildings intuitively cater to the people inside them, creating dynamic, human-centric environments.
Conclusion: A Paradigm Shift in Building Design
Machine learning in thermal comfort isn’t just a technological advance—it’s a rethinking of how we design spaces. By moving beyond static, one-size-fits-all solutions, we’re embracing a future of responsive, energy-efficient buildings that genuinely enhance human experience.
For facility managers, system integrators, and building owners, this represents more than an upgrade. It’s a call to action to redefine the relationship between humans and their environments—making comfort personal, sustainable, and undeniably smart.